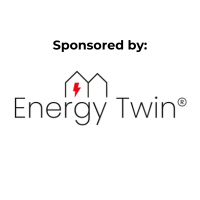
Finding Black Sheep in your Building Portfolio Using Explainable AI
Abstract:
AI is transforming energy management, offering Energy Managers, TAB professionals, Cx agents, and Facility Managers powerful tools for efficiency and cost reduction. This presentation highlights practical, data-driven applications that reveal energy-saving opportunities, optimize cooling, and reduce operational waste across individual buildings and large portfolios.
Attendees will explore real-world examples, including a fast-food chain case study demonstrating how these solutions yield measurable savings and operational improvements.
Learning Objectives:
- Understanding Key Challenges in Developing Meaningful Machine Learning Models for Energy Management
- Leveraging Explainable AI to Generate Actionable Insights for Energy Savings
- Exploring Anomaly Detection Techniques and Their Impact on Energy Efficiency
- Scalability and Cost-Effectiveness of AI Solutions for Large Building Portfolios
Sponsored by: Energy Twin
Energy Twin specializes in advanced machine learning solutions for energy management. Beyond its primary offerings for building portfolio analysis, the company provides customized machine learning solutions tailored to specific client needs, enabling diverse organizations to optimize energy consumption and reduce operational costs. With a focus on scalability and efficient energy use, Energy Twin enables clients to drive impactful energy savings through accessible, data-driven insights.
Speakers
-
Allison FitzpatrickCo-Founder, ACTuate Facility Technologies
Allison Fitzpatrick is the Cofounder of ACTuate Facility Technologies, an energy services company specializing in multi-location building portfolios. Allison has over 15 years of experience in energy efficiency and renewables within the built environment, with a focus on energy and IoT data analytics. Allison earned a Bachelor of Science degree in Physics from Elon University.
-
Jan ŠirokýEnergy Twin CEO
Jan Široký, PhD, founder and CEO of Energy Twin, leverages over two decades of expertise in energy data analysis and building control systems. With a PhD in active fault detection and a strong research background, Jan drives Energy Twin’s AI-powered solutions for optimizing energy consumption in buildings. He has collaborated with universities on multiple research projects in AI-driven energy management and fault detection, advancing intelligent, sustainable building systems. His research has been published in leading journals like Applied Energy and Energy and Buildings, and he has co-authored studies on model predictive control and energy-efficient heating systems.